Human-AI
Interaction Lab
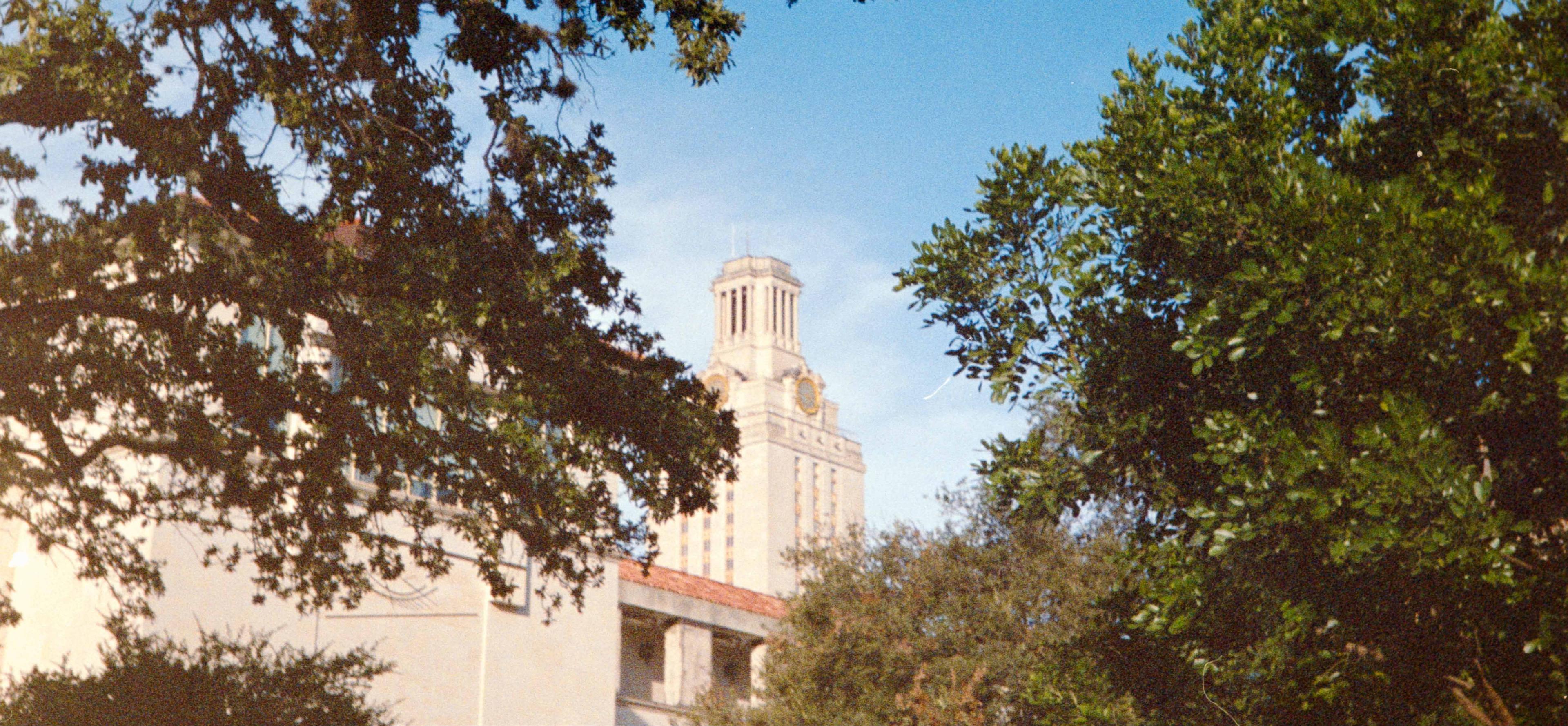
Our Mission
Building more just and empowering workplaces and cities by creating technology that supports and strengthens individual and collective human decision-making.
Highlights
Data Probes as Boundary Objects for Technology Policy Design: Demystifying Technology for Policymakers and Aligning Stakeholder Objectives in Rideshare Gig Work
Despite the evidence of harm that technology can inflict, commensurate policymaking to hold tech platforms accountable still
lags. This is pertinent to app-based gig workers, where unregulated algorithms continue to dictate their work, often with little
human recourse. While past HCI literature has investigated workers’ experiences under algorithmic management and how to design
interventions, rarely are the perspectives of stakeholders who inform or craft policy sought. To bridge this, we propose using data
probes—interactive visualizations of workers’ data that show the impact of technology practices on people—exploring them in 12 semistructured interviews with policy informers, (driver-)organizers,
litigators, and a lawmaker in the rideshare space. We show how
data probes act as boundary objects to assist stakeholder interactions, demystify technology for policymakers, and support worker
collective action. We discuss the potential for data probes as training tools for policymakers, and considerations around data access
and worker risks when using data probes.
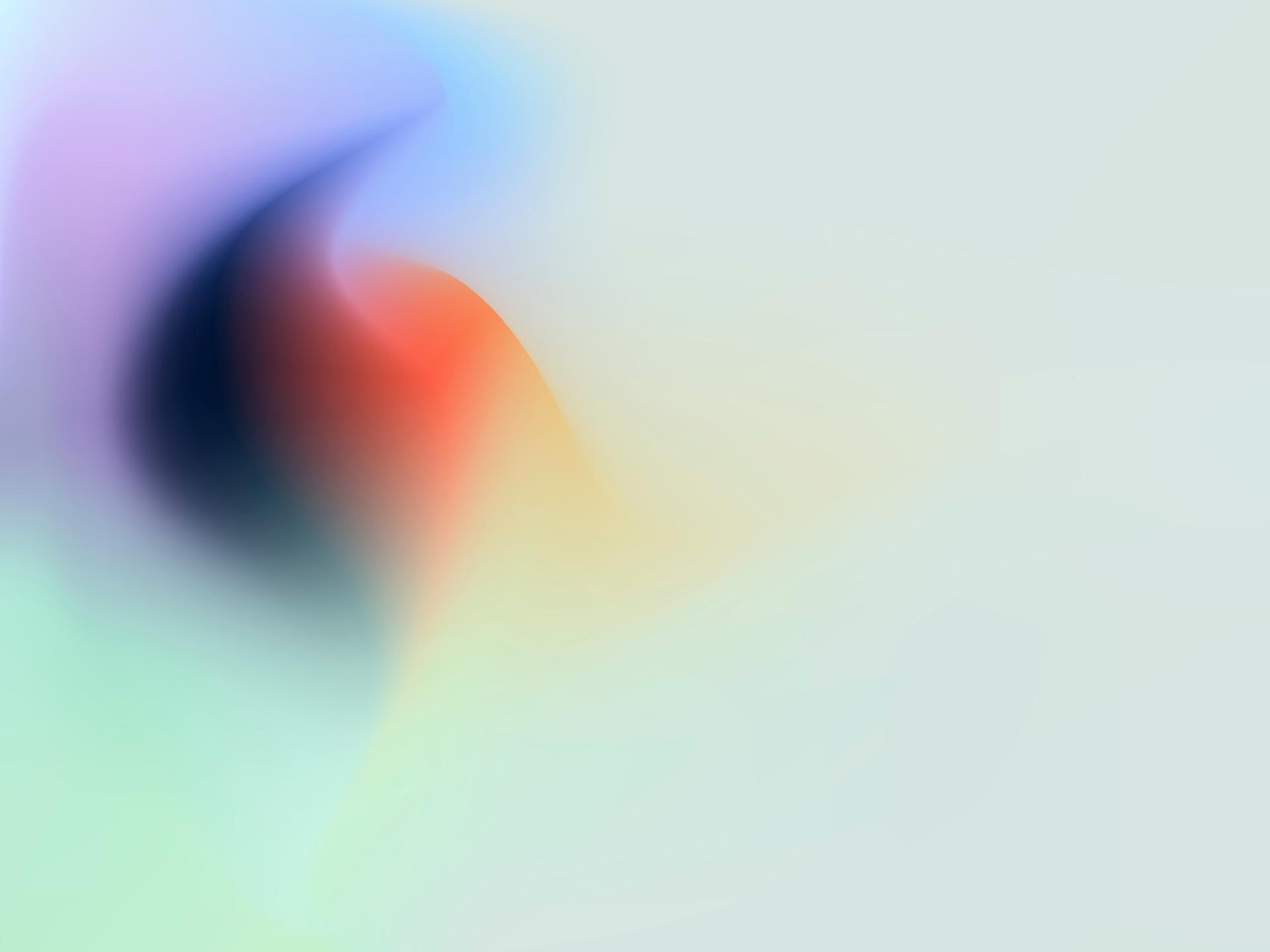
Aligning Data with the Goals of an Organization and Its Workers: Designing Data Labeling for Social Service Case Notes
The challenges of data collection in nonprofits for performance and
funding reports are well-established in HCI research. Few studies,
however, delve into improving the data collection process. Our
study proposes ideas to improve data collection by exploring challenges that social workers experience when labeling their case
notes. Through collaboration with an organization that provides
intensive case management to those experiencing homelessness
in the U.S., we conducted interviews with caseworkers and held
design sessions where caseworkers, managers, and program analysts examined storyboarded ideas to improve data labeling. Our
findings suggest several design ideas on how data labeling practices
can be improved: Aligning labeling with caseworker goals, enabling
shared control on data label design for a comprehensive portrayal
of caseworker contributions, improving the synthesis of qualitative and quantitative data, and making labeling user-friendly. We
contribute design implications for data labeling to better support
multiple stakeholder goals in social service contexts.
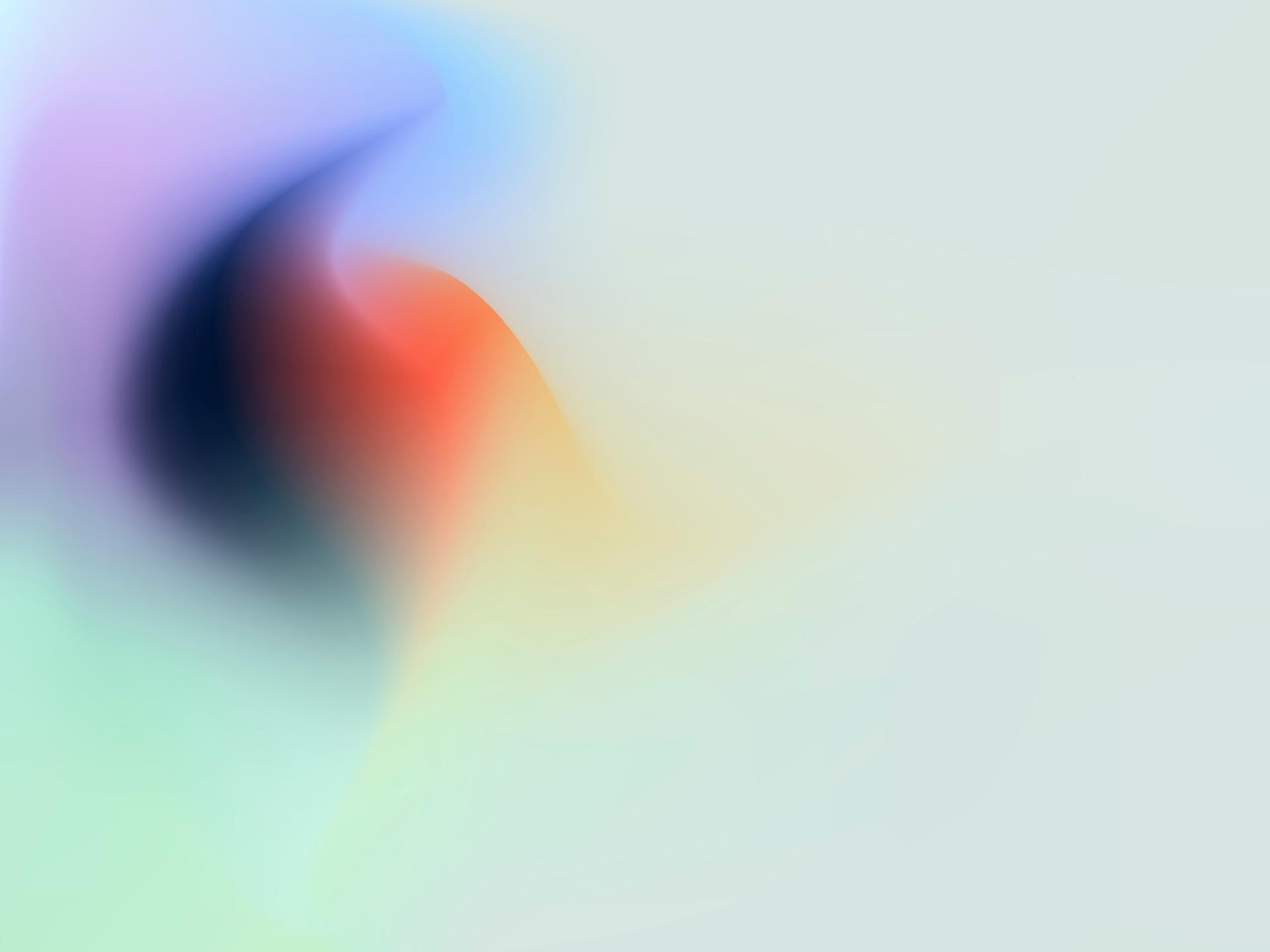
Current Projects
Reimagining Algorithmic Management for Worker Well-Being through Worker Co-Design and Policy Approaches
Increasingly, people are turning to gig work platforms for flexible work opportunities, yet paradoxically, research has shown that algorithmic management controls gig workers through tactics such as gamified incentives and opaque work assignment and commission rates. We use co-design methods to engage with stakeholders—e.g., workers, organizers, and policymakers—to understand how algorithmic management impacts worker well-being, surface ideas for interventions to support worker protections, and design tools to help with policymaking. We are currently designing policymaking training sessions to illustrate to policymakers what algorithmic management is and how it impacts workers and to surface policy needs—e.g., specific wording to use in bills, and data needed to help garner colleague support. We are also collaborating with partners at CMU and UMN to prototype a data-sharing system for gig workers and policymakers to investigate worker issues and inform related policies.
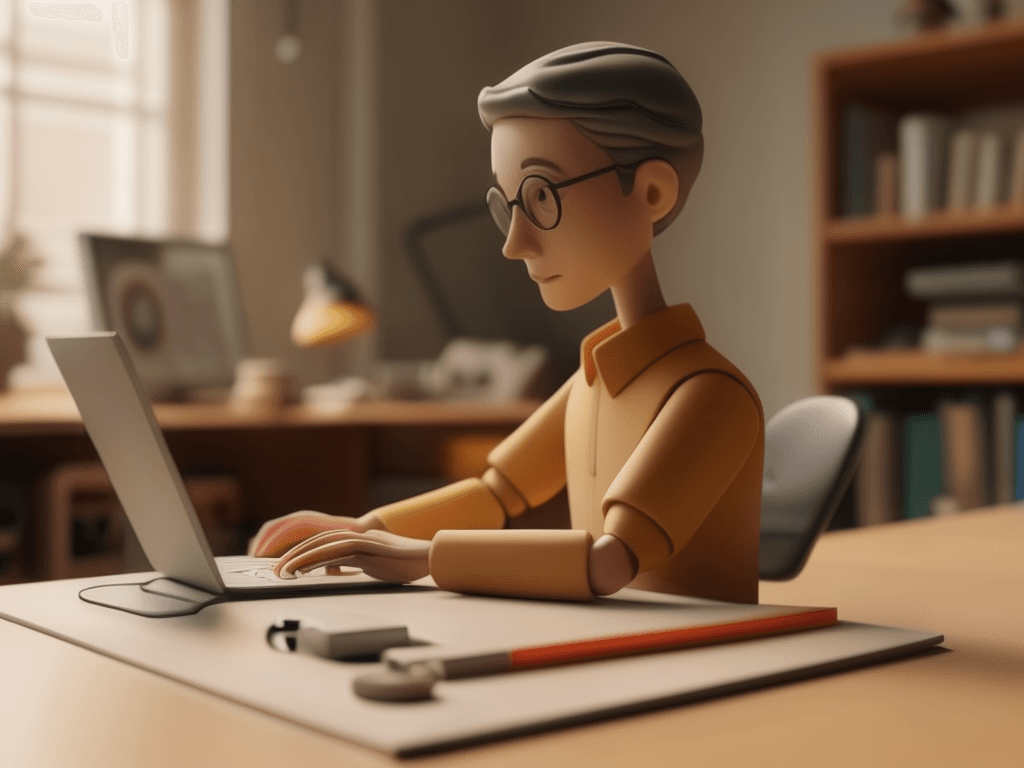
Designing Tools to Support Organizational Decision-Making and Support Participatory AI Design
One concern of AI technologies is its potential to generate inequitable outcomes for underrepresented populations, often linked to overlooked historical human biases within datasets or lack of input from impacted constituents. This has led to increased calls eschewing AI automation for AI assistance. Yet, AI assistance still encompasses challenges including 1) humans drawing on AI assistance can still exhibit biased decision-making, and 2) in organizations, it can be unclear how to resolve differences in what values to embed in AI assistive tools. To that end, we explored how to support organizational deliberation to support more inclusive outcomes through focus groups where participants used historical data to construct personal models about Master’s admissions and surface patterns of organizational decision-making to inform future practices. Based on how participants used their personal ML models as boundary objects to deliberate together and share situational contexts informing their preferences, we are currently exploring how to systematically integrate impacted stakeholder participation in the data exploration phase of AI design for whether this can surface diverse perspectives for (un)acceptable uses of data.
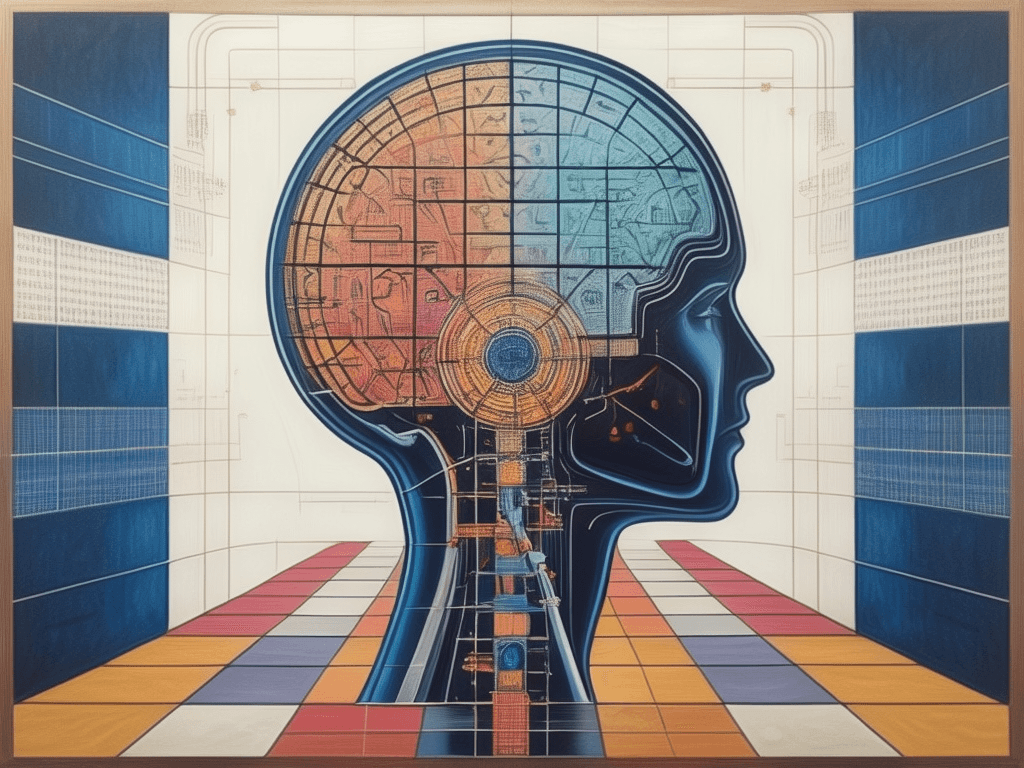
News and Announcements
Apr 2024
Dr. Lee and Angie Zhang are delivering a talk as part of the William Pierson Field Lecture initiative at Princeton.
Apr 2024
Angie Zhang is co-organizing a 2024 FAccT tutorial titled "Navigating Equity and Reflexive Practices in Gigwork Design—A Journey Mapping Experience"
Apr 2024
Dr. Lee is giving a keynote at the UMich Annual Ethical AI Symposium
Jan 2024