Current Projects
This is what we’re working on right now
Reimagining Algorithmic Management for Worker Well-Being through Worker Co-Design and Policy Approaches
Increasingly, people are turning to gig work platforms for flexible work opportunities, yet paradoxically, research has shown that algorithmic management controls gig workers through tactics such as gamified incentives and opaque work assignment and commission rates. We use co-design methods to engage with stakeholders—e.g., workers, organizers, and policymakers—to understand how algorithmic management impacts worker well-being, surface ideas for interventions to support worker protections, and design tools to help with policymaking. We are currently designing policymaking training sessions to illustrate to policymakers what algorithmic management is and how it impacts workers and to surface policy needs—e.g., specific wording to use in bills, and data needed to help garner colleague support. We are also collaborating with partners at CMU and UMN to prototype a data-sharing system for gig workers and policymakers to investigate worker issues and inform related policies.
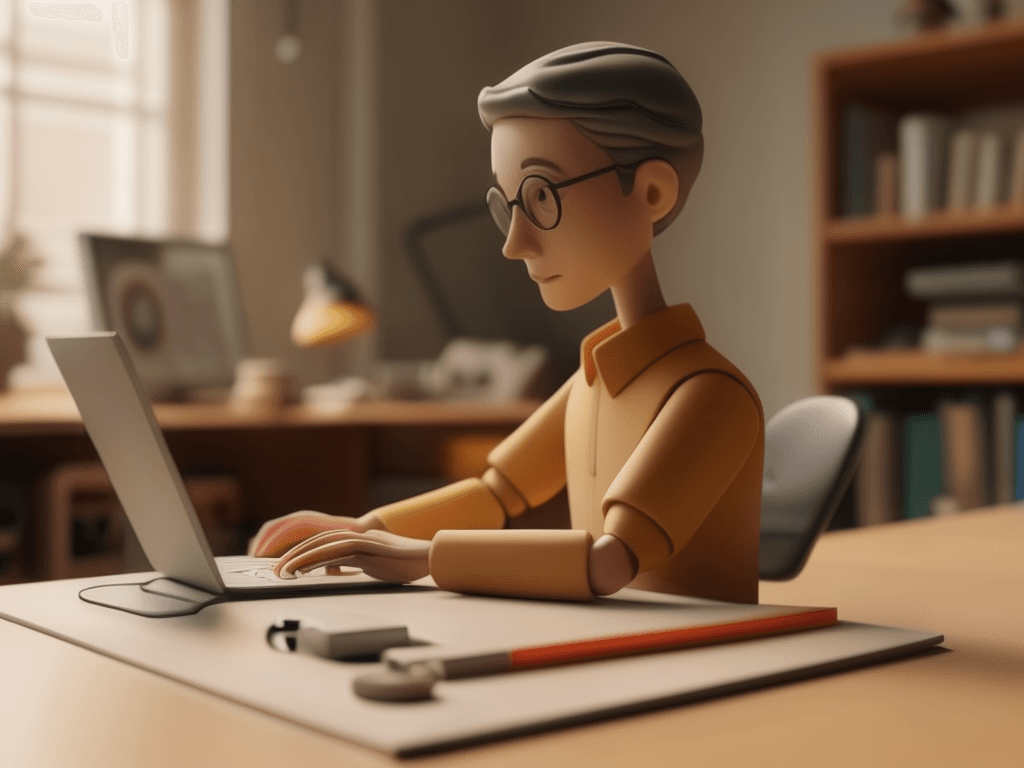
Designing Tools to Support Organizational Decision-Making and Support Participatory AI Design
One concern of AI technologies is its potential to generate inequitable outcomes for underrepresented populations, often linked to overlooked historical human biases within datasets or lack of input from impacted constituents. This has led to increased calls eschewing AI automation for AI assistance. Yet, AI assistance still encompasses challenges including 1) humans drawing on AI assistance can still exhibit biased decision-making, and 2) in organizations, it can be unclear how to resolve differences in what values to embed in AI assistive tools. To that end, we explored how to support organizational deliberation to support more inclusive outcomes through focus groups where participants used historical data to construct personal models about Master’s admissions and surface patterns of organizational decision-making to inform future practices. Based on how participants used their personal ML models as boundary objects to deliberate together and share situational contexts informing their preferences, we are currently exploring how to systematically integrate impacted stakeholder participation in the data exploration phase of AI design for whether this can surface diverse perspectives for (un)acceptable uses of data.
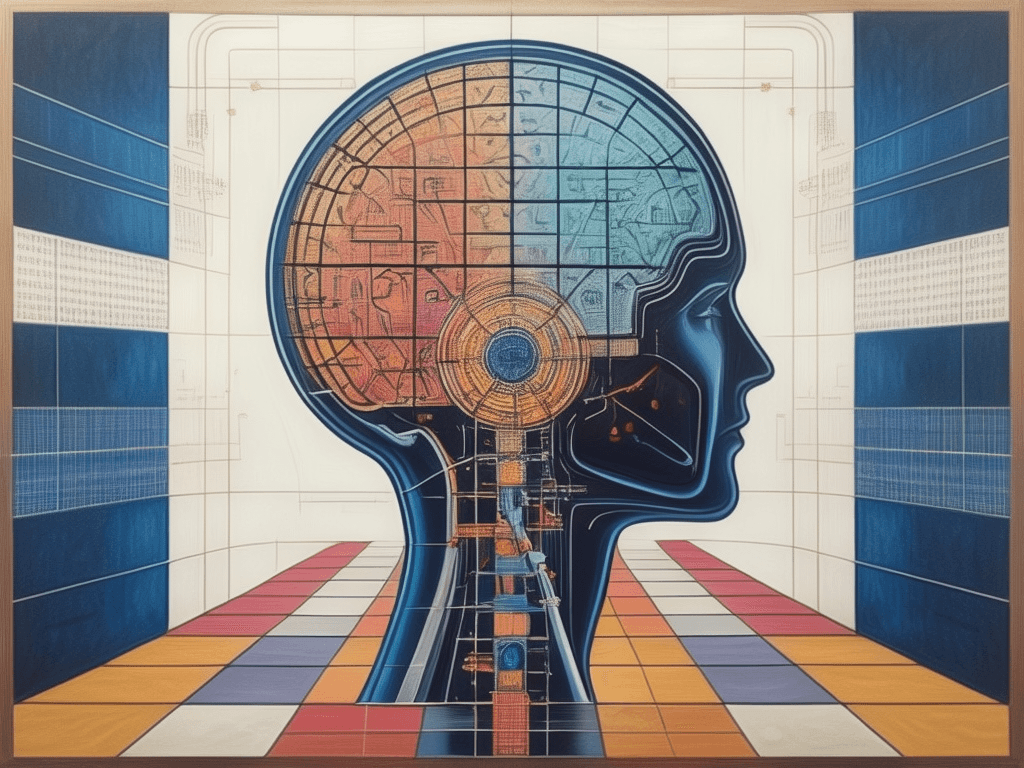
Applying an Equity Lens to Understand Public Administration Challenges and Needs around Data and Technology Use
Across the U.S., city governments are establishing equity initiatives to address systemic inequities. Yet, relatively little work has investigated how governments are grappling with equity and the impact of their technology practices on equitable public administration. To study this gap, we turn to the longstanding pursuit of equity by public administration as a lens of inquiry for how to design public sector tech and data-driven processes. We are currently interviewing employees of two different U.S. local governments to learn how centering their equity practices and experiences can expand our understanding for how to design acceptable and equitable government data-driven systems and technology.
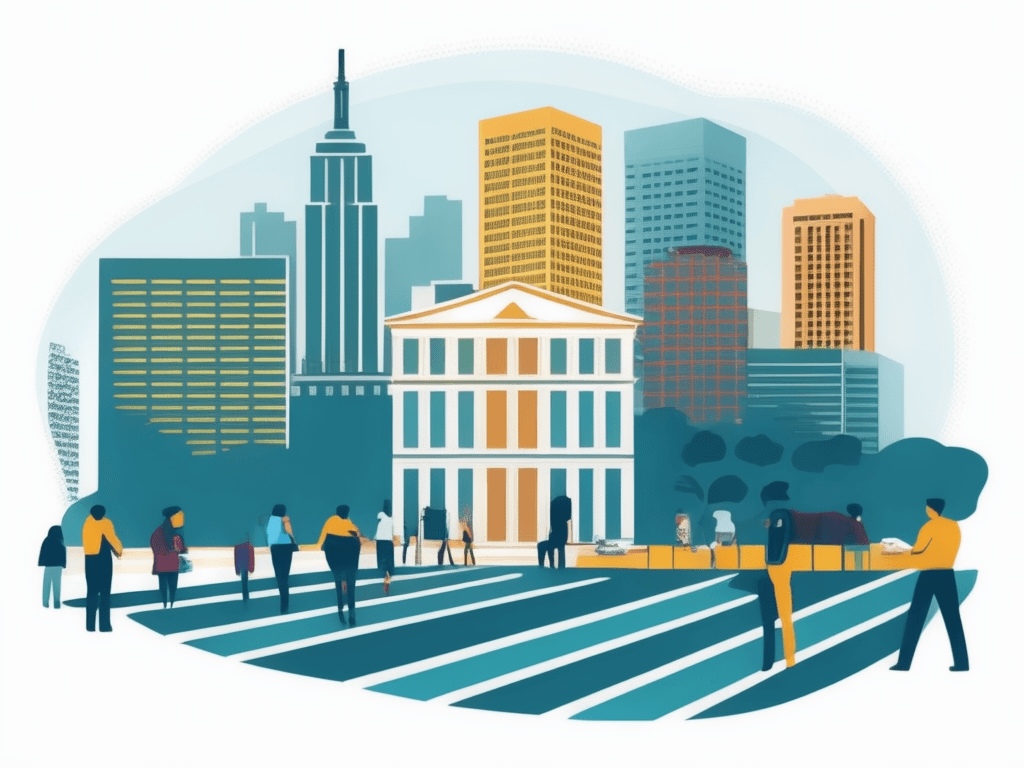
Making Algorithmic Work Management Software Accountable to Law
Software systems have become an integral part of public and private sector management, yet alarming evidence suggests that they often significantly undermine workforce well-being and are not accountable to existing labor laws. Still, there is not yet an established mechanism to encode law into software, and protections for people affected by these new software systems under existing laws are often inadequate. To tackle these challenges, in collaboration with Harvard, UConn, and UCSD, we employ participatory methods to understand the situated practices and preferences of the diverse stakeholders who both regulate and use these software systems. We also aim to make these software accountable to law and policy, and leverage it to make software users (individuals and firms) accountable to the affected population and the public.
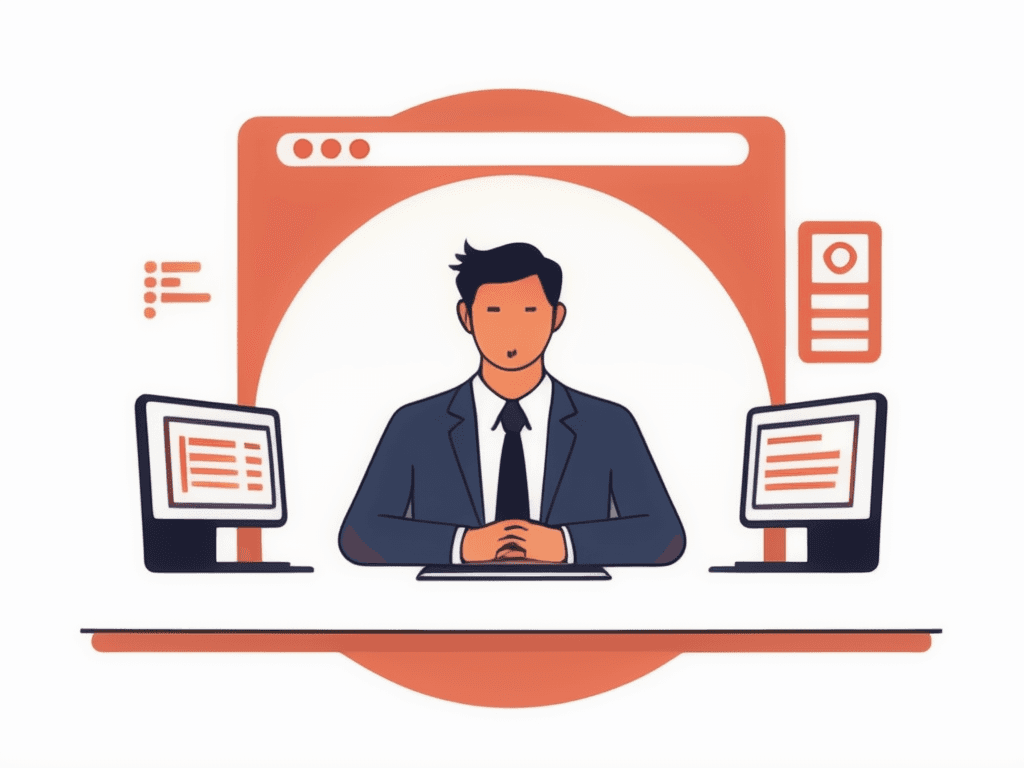
Participatory Algorithmic Governance in the Non-Profit Sector
Algorithms are increasingly being incorporated into the service-provision processes of organizations that support the needs of the community. But how can we design these algorithmic services to make decisions in a way that not only meets these organizations’ operational needs but can also balance diverse and varying stakeholder interests in a fair and legitimate way? In partnership with an international food rescue organization, we present a collective participatory framework that enables people to build algorithmic policies for their communities so that the algorithmic decisions align with their values and needs. We are also exploring and evaluating methods to scale this participatory framework so that efforts that seek to bring these tools to a broader audience remain aligned to the interests, goals within each local context.
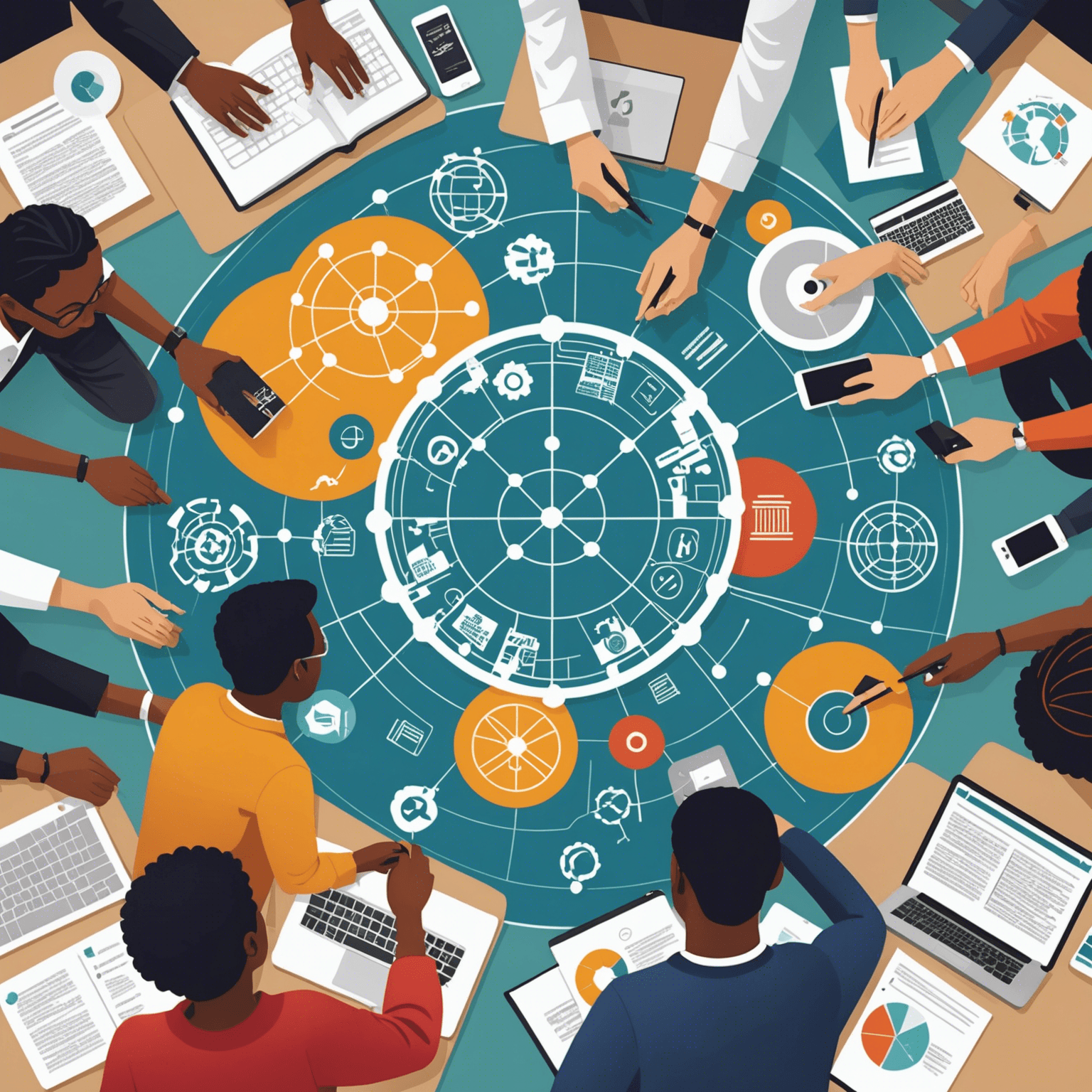
Community Driven Urban Air Mobility: Multi-stakeholder Deliberation for Urban AI
City administrators worldwide are seeking to leverage AI to improve urban planning and operations. However, they must be mindful of the potential risks to equity and community representation, as AI could become a proxy for community values. One area of interest for urban planners is future transportation infrastructure. With this in mind, designing AI tools and systems that accurately reflect community values and incorporate a multi-stakeholder perspective is crucial. To study this, we are utilizing co-design methods and drawing on theories of multi-stakeholder deliberation and mini-publics. We work in co-design focus groups with community organizations to gain insight into their transportation-related values and perceptions of AI tools in urban planning and operations.
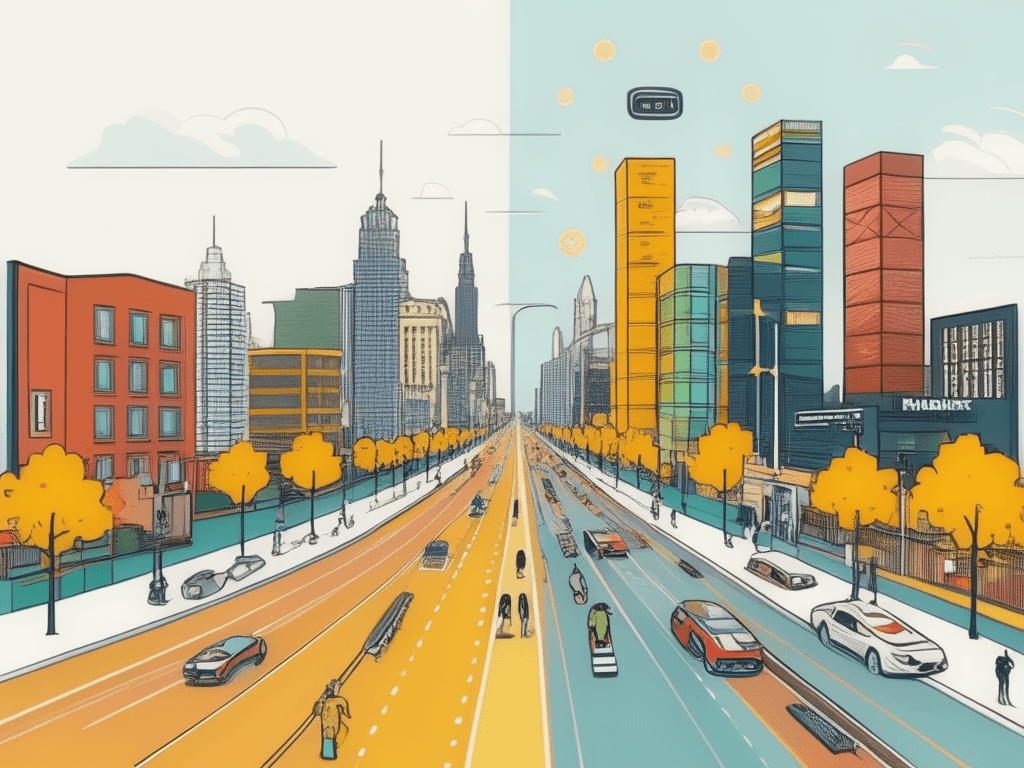
Designing Human-Centered AI for Homelessness Caseworkers: Aligning data collection goals and exploring LLM based solutions
Social workers are required to collect data for financial and performance reporting. Through free text case notes and pre-defined data labels, caseworkers provide invaluable information on their organization's impact on the community. However, caseworkers view data collection as an additional burden that does not contribute to their ability to serve clients. By taking a human-centered, co-design approach, we explore how caseworker goals can align with data collection and be used to improve their interactions with clients. We have previously explored data labeling solutions through the speed dating methodology. We are currently exploring LLM-based design solutions that center on human interaction. Our focus is on capabilities that can upskill caseworker practices, rather than deskill casework.
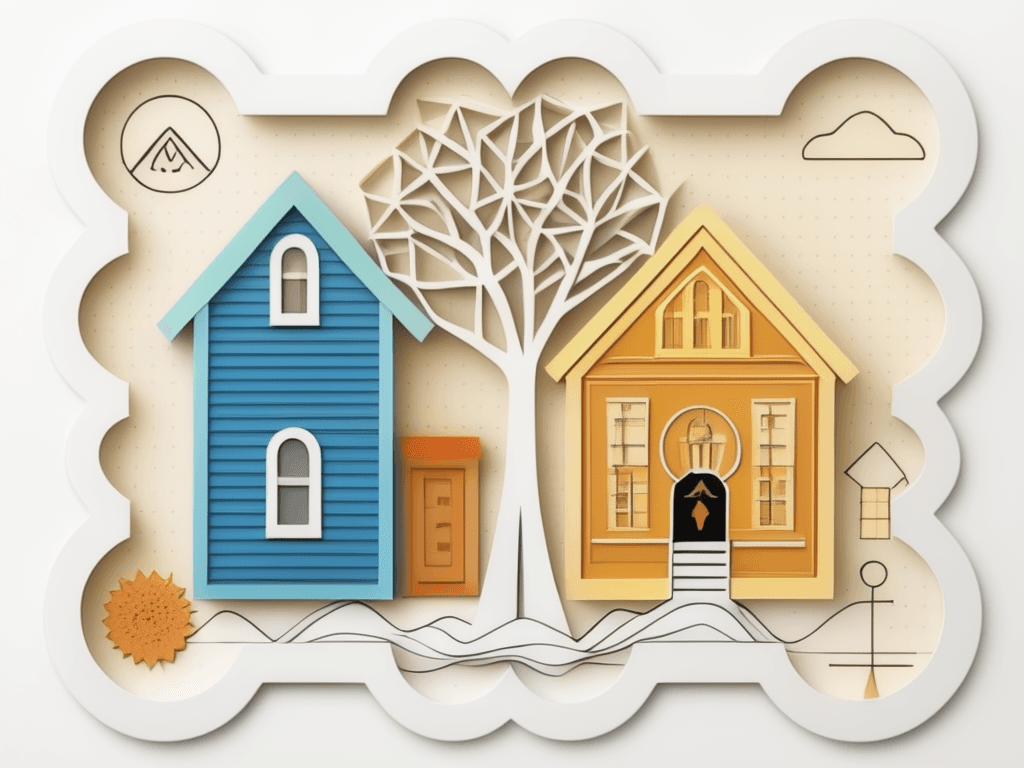